Philosophy
Interpretations of Probability
Nov 2, 2022
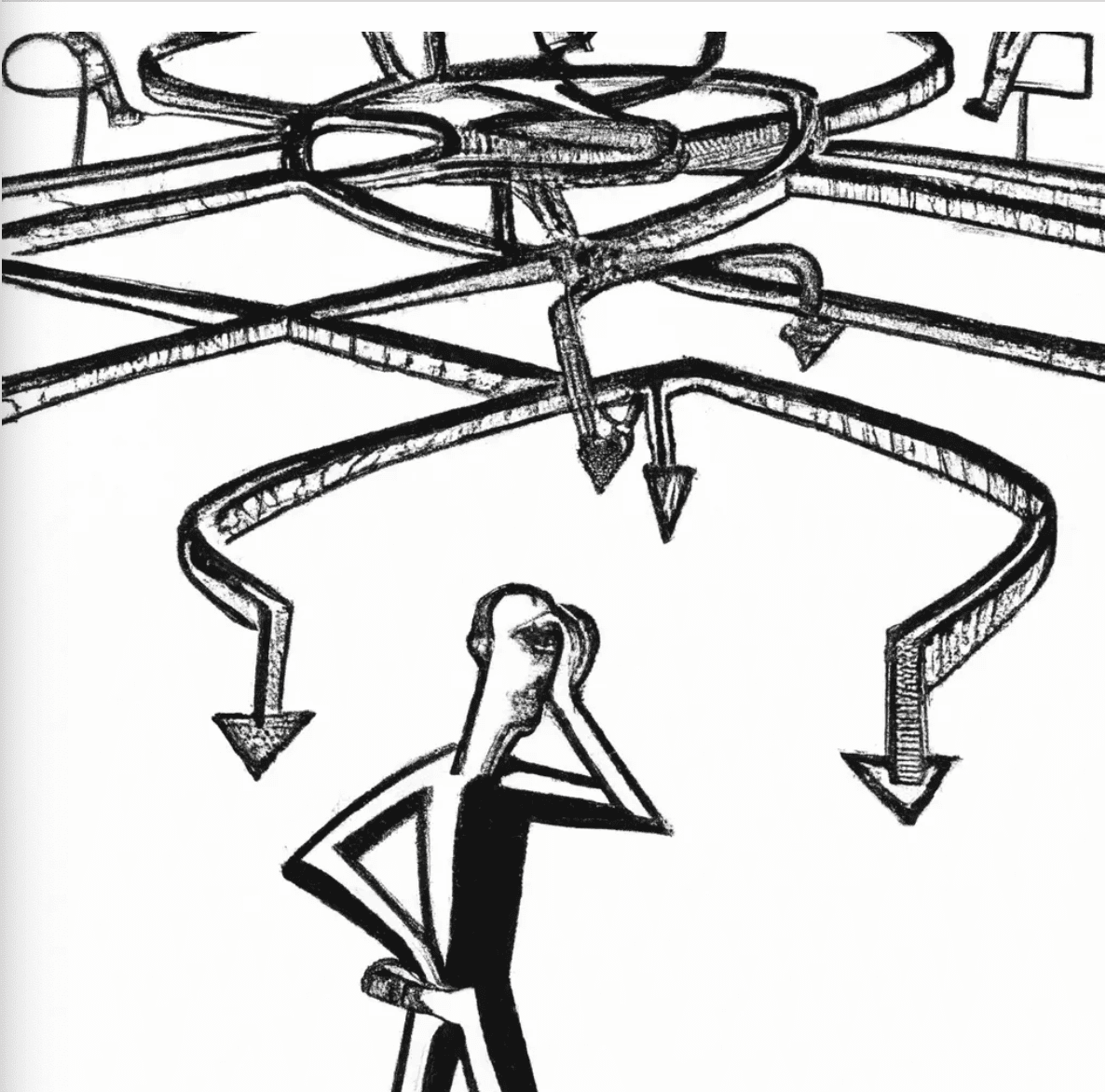
Probability is the most important concept in modern science, especially as nobody has the slightest notion what it means.—Bertrand Russell, 1929
Probabilities are everywhere. They’re used in everything from game theory and gambling to weather forecasting, epidemiology and evolutionary biology. Despite their ubiquity, the answer to the question “what, fundamentally, is probability?” is deceptively tricky. I will attempt to unpack some of the leading interpretations of probability and hopefully, get you a little closer to tackling this question.
At its most basic level, probability is just a function. It takes an input (in this case, events or propositions) and spits out a real number between 0 and 1 that is supposed to measure the likelihood of that input. The rules that define this process are called the Kolmogorov Axioms and they tell us some pretty straightforward things about how this function behaves. At this level, there’s nothing really complicated or controversial about probability. After all, it’s just a number. Things get a little dicier when we try to apply this function to things in the real world.
Let’s say I’m a student doing my A-levels and I’m thinking about whether or not I should submit one of my papers for remarking. A natural question I’d ask is, ‘what is the probability that my mark changes after submitting it for remarking?’. One way to go about answering this question is to gather the following information from the exam board:
How many papers were submitted for remarking in the last five years?
How many papers that were submitted for remarking had their marks changed?
Divide 2 by 1 and you’re done! You now have a probability of getting your mark changed.

If this method seems familiar to you, that’s probably because it illustrates an interpretation of probability that is most commonly taught in statistics classes and is known as the frequentist approach to probability. In this view, probability is the relative frequency of some attribute compared to some reference class. in our example, probability is the relative frequency of papers that had their marks changed compared to the number of papers submitted for remarking. This interpretation seems intuitive, practical and grounded in reality. However, things get a bit weird when you consider some alternative cases that aren’t this straightforward. Say I asked you to flip a fair coin 17 times and use the frequentist approach to tell me what the probability of the coin landing heads is. In this case, it is totally plausible (albeit unlikely) that you flip the coin 17 times and it lands on heads 12 times and tails 5 times. The frequentist approach would lead you to conclude that the probability of a fair coin landing heads is 12/17. That’s weird. Everyone knows it should be 1/2! In fact, if we were to use this particular frequentist approach where we merely count the relative frequencies and calculate a ratio, it is impossible for us to get a probability of 1/2 because we’re flipping the coin an odd number of times. This method where we just count and calculate a ratio is known as the finite frequentist approach. Another way to be a frequentist that avoids this problem is to say that probabilities are limiting relative frequencies. In other words, the probability that a fair coin lands heads is the relative frequency of a coin landing heads if I were to flip the coin an infinite number of times. By the Law of Large Numbers, so the theory states, this ratio should converge to the true probability (1/2). This method is known as hypothetical frequentism and, although it also seems pretty straightforward, it is a little more abstract and less grounded in reality.
Both of these approaches treat probability as an objective property of the world around us - it is completely independent of people’s beliefs about it. This works just fine for most things we need probabilities for and indeed, most statistics and scientific research employs a frequentist approach to probabilities. But what happens if I were to ask you, ‘What is the probability that an all-out nuclear war results in human extinction?’ or, ‘Suppose I’m asked to pick any real number. What is the probability that I pick the number 7?’. In these cases, the frequentist approach is woefully insufficient. Why? Take the first case. The frequentist would tell us that we need to find the relative frequency of humanity-ending nuclear war. The problem is, there has never been such a war. If there was, you wouldn’t be reading this article. Humanity-ending nuclear war is an unrepeatable event so we can’t use the frequentist approach to calculate this probability. In the second case, the problem isn’t that we don’t have enough repeatable events to use as evidence. The problem is that we have too many. We could write this probability as 1 / (the number of real numbers), assuming some principle of indifference. The problem is that there are infinitely many real numbers so this ratio is undefined! So what do we do in cases like these? It seems strange to give up and refuse to assign probabilities to these kinds of events or propositions. Here’s where the Bayesian interpretation of probabilities comes in.
Under the Bayesian view, probabilities are ways of quantifying your degrees of belief about events. On this view, stating that P( ‘all-out nuclear war results in human extinction’ ) = 0.85 corresponds to saying ‘I believe that there’s an 85% chance that all-out nuclear war results in human extinction.’ This is useful because it allows us to form probabilities about all kinds of propositions that were just not possible under the frequentist approach. Like with relative frequencies, the method for calculating probabilities under the Bayesian view is given by Bayes rule.
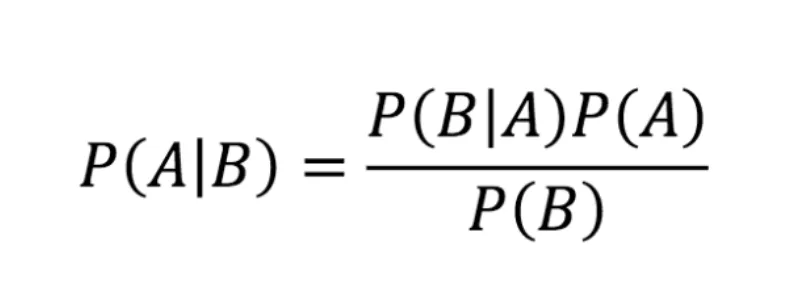
Bayes rule is cool enough to warrant its own segment in a future newsletter but for now, the thing to note about it is that it relies on a process of conditionalisation to generate probabilities. Since probabilities under Bayesianism represent ‘how much I believe in some event or proposition’, we can develop a theory of how your beliefs change in response to new evidence. For example, my belief that it is raining outside, P(Rain) is higher if I see people outside taking out their umbrellas than if I see people outside taking out their sunglasses. ie: P(Rain | Umbrellas) > P(Rain | Sunglasses). This view of probabilities, like the frequentist view, is constrained by the Kolmogorov axioms but unlike the frequentist view, the constraints may stop there. When I first learned about Bayesianism, it blew my mind. There was a completely new and different way of thinking about probabilities that opened up so many interesting avenues for developing my models of the world.
What’s really interesting about these different views of probability is that they both refer to the same fundamental mathematical object but offer vastly different philosophical interpretations that have pretty big implications for how we think about randomness, beliefs and making decisions under uncertainty.
Click here to learn more about the Bayes rule, here and here to read about problems with finite and hypothetical frequentism (respectively).